Towards a Pipeline for Real-Time Visualization of Faces for VR-based Telepresence and Live Broadcasting Utilizing Neural Rendering
DOI:
https://doi.org/10.48663/1860-2037/18.2024.1Keywords:
Telepresence, Neural Rendering, Face Reconstruction, Virtual Reality, Live Broadcasting, Image-to-Image Translation, Pix2Pix, Generative Adversarial NetworksAbstract
While head-mounted displays (HMDs) for Virtual Reality (VR) have become widely available in the consumer market, they pose a considerable obstacle for realistic face-to-face conversation in VR since HMDs hide a significant portion of the participants faces. Even with image streams from cameras directly attached to an HMD, stitching together a convincing image of an entire face remains a challenging task because of extreme capture angles and strong lens distortions due to a wide field of view. Compared to the long line of research in VR, reconstruction of faces hidden beneath an HMD is a very recent topic of research. While the current state-of-the-art solutions demonstrate photo-realistic 3D reconstruction results, many of them require high-cost laboratory equipment and large computational costs. We present an approach that focuses on low-cost hardware and can be used on a commodity gaming computer with a single GPU. We leverage the benefits of an end-to-end pipeline by means of Generative Adversarial Networks (GAN). Our GAN produces a frontal-facing 2.5D point cloud based on a training dataset captured with an RGBD camera. In our approach, the training process is offline, while the reconstruction runs in real-time. Our results show adequate reconstruction quality within the “learned” expressions. Expressions not learned by the network produce artifacts and can trigger the Uncanny Valley effect.
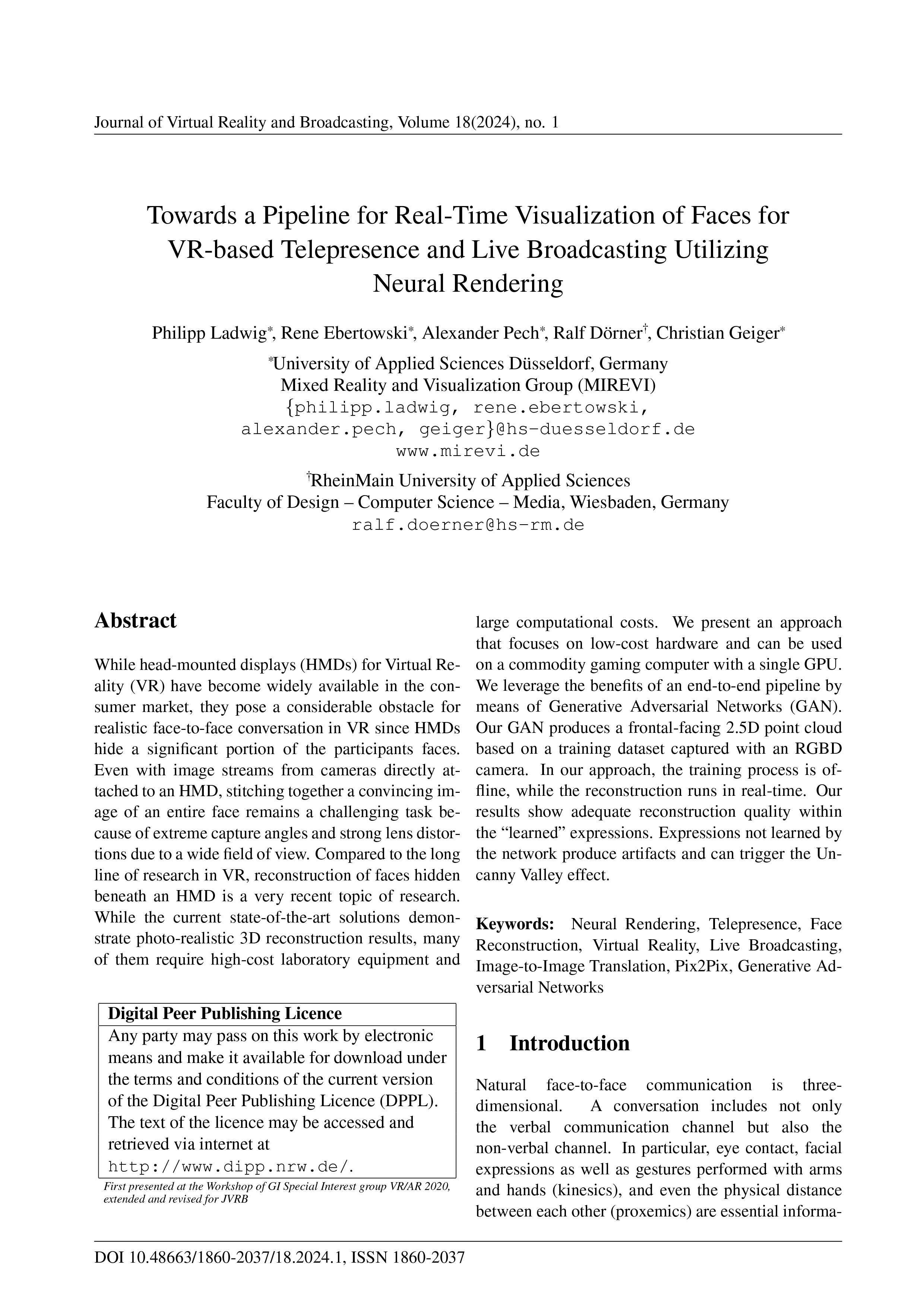